Lots of interesting abstracts and cases were submitted for TCTAP 2023. Below are the accepted ones after a thorough review by our official reviewers. Don¡¯t miss the opportunity to expand your knowledge and interact with authors as well as virtual participants by sharing your opinion in the comment section!
TCTAP A-086
Can a Machine Learning Based Approach Improve Risk Prediction and Individualise Decision Making Between Percutaneous and Surgical Revascularisation in Patients With Complex Coronary Artery Disease?
By Kai Ninomiya, Shigetaka Kageyama, Hiroki Shiomi, Shinichiro Masuda, Nozomi Kotoku, Pruthvi Revaiah, Scot Garg, Neil O¡¯Leary, Takeshi Kimura, Yoshinobu Onuma, Patrick W. Serruys
Presenter
Kai Ninomiya
Authors
Kai Ninomiya1, Shigetaka Kageyama2, Hiroki Shiomi3, Shinichiro Masuda2, Nozomi Kotoku2, Pruthvi Revaiah2, Scot Garg4, Neil O¡¯Leary2, Takeshi Kimura5, Yoshinobu Onuma2, Patrick W. Serruys2
Affiliation
Iwate Medical University, Japan1, University of Galway, Ireland2, Kyoto University, Japan3, Royal Blackburn Hospital, United Kingdom4, Hirakata Kohsai Hospital, Japan5
View Study Report
TCTAP A-086
Others (Unclassified)
Can a Machine Learning Based Approach Improve Risk Prediction and Individualise Decision Making Between Percutaneous and Surgical Revascularisation in Patients With Complex Coronary Artery Disease?
Kai Ninomiya1, Shigetaka Kageyama2, Hiroki Shiomi3, Shinichiro Masuda2, Nozomi Kotoku2, Pruthvi Revaiah2, Scot Garg4, Neil O¡¯Leary2, Takeshi Kimura5, Yoshinobu Onuma2, Patrick W. Serruys2
Iwate Medical University, Japan1, University of Galway, Ireland2, Kyoto University, Japan3, Royal Blackburn Hospital, United Kingdom4, Hirakata Kohsai Hospital, Japan5
Background
In patients with three-vessel coronary artery disease (CAD) and/or left main (LM) CAD, individual risk prediction plays a key role in deciding between percutaneous coronary intervention (PCI) and coronary artery bypass grafting (CABG). The aim of this study was to assess whether these individualised revascularization decisions can be improved by applying machine learning (ML) algorithms and integrating clinical, biological, and anatomical factors.
Methods
In the SYNTAXES study, ML algorithms (Lasso regression, gradient boosting) were used to develop a prognostic index for 5-year death, which was combined, in the second stage, with assigned treatment (PCI or CABG), and pre-specified effect-modifiers: disease type (3VD or LMCAD) and anatomical SYNTAX score. The model¡¯s discriminative ability to predict the risk of 5-year death and treatment benefit between PCI and CABG was cross-validated in the SYNTAX trial (n=1800 patients) and externally validated in the CREDO-KYOTO registry (n=7362), and then compared to the original SYNTAX score II 2020 (SS2020).
Results
The gradient boosting model performed best for predicting 5-year all-cause death with C-indexes of 0.78 (95% CI 0.75-0.81) in cross-validation and 0.77 (95% CI 0.76-0.79) in external validation. The ML models discriminated 5-year mortality better than the SS2020 in the external validation cohort and identified heterogeneity in the treatment benefit of CABG versus PCI (Figure).
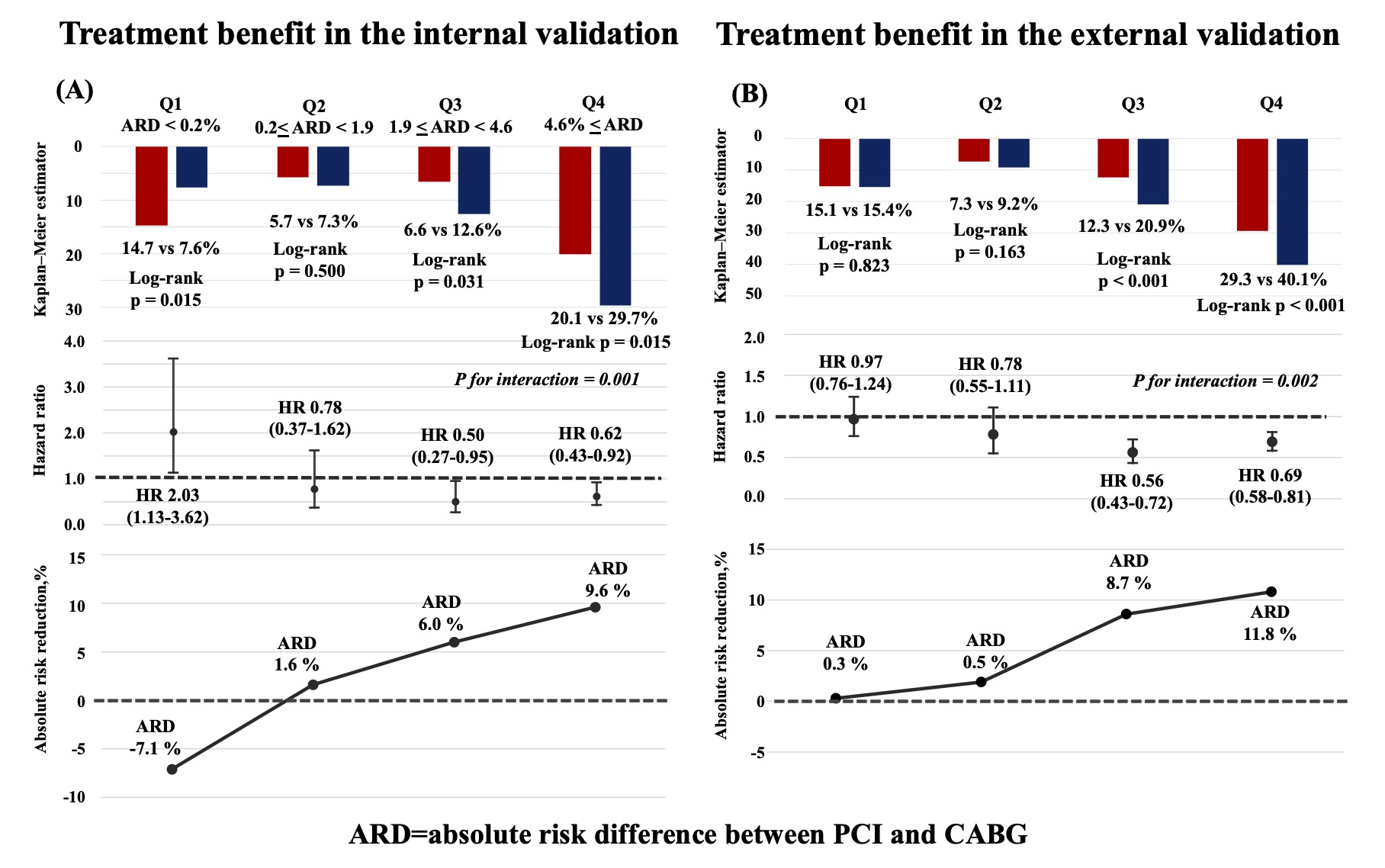
Conclusion
An ML-based approach for identifying individuals who benefit from CABG or PCI is feasible and effective. Implementation of this model in health care systems—trained to collect large numbers of parameters—may harmonize decision-making globally.