Lots of interesting abstracts and cases were submitted for TCTAP 2022. Below are the accepted ones after a thorough review by our official reviewers. Don¡¯t miss the opportunity to expand your knowledge and interact with authors as well as virtual participants by sharing your opinion in the comment section!
TCTAP A-074
Quantifying Coronary Arterial Stenosis and Measuring the Arterial Phase by Artificial Intelligence Program
By Loc Vu, Thai Dien Pham, Tri Tai Truyen, Quang Minh Mai, Thuan Huynh, Trung Thong Hoang, My Linh Phan Khanh, Cam Tu Tran Thi, Dung Ho, Thach Nguyen
Presenter
Tri Loc Vu
Authors
Loc Vu1, Thai Dien Pham1, Tri Tai Truyen1, Quang Minh Mai1, Thuan Huynh2, Trung Thong Hoang3, My Linh Phan Khanh1, Cam Tu Tran Thi1, Dung Ho4, Thach Nguyen5
Affiliation
Tan Tao University, Vietnam1, University of Medicine and Pharmacy at Ho Chi Minh City, Vietnam2, Military Hospital 175, Vietnam3, Thong Nhat Hospital, Vietnam4, Methodist Hospital, Meriville, USA5
View Study Report
TCTAP A-074
Physiologic Lesion Assessment
Quantifying Coronary Arterial Stenosis and Measuring the Arterial Phase by Artificial Intelligence Program
Loc Vu1, Thai Dien Pham1, Tri Tai Truyen1, Quang Minh Mai1, Thuan Huynh2, Trung Thong Hoang3, My Linh Phan Khanh1, Cam Tu Tran Thi1, Dung Ho4, Thach Nguyen5
Tan Tao University, Vietnam1, University of Medicine and Pharmacy at Ho Chi Minh City, Vietnam2, Military Hospital 175, Vietnam3, Thong Nhat Hospital, Vietnam4, Methodist Hospital, Meriville, USA5
Background
Coronary angiography (CAG) plays a crucial role in diagnosing coronary artery disease (CAD) by identifying the vessel size, location, and percentage of stenosis. This is the current conventional modality used by human investigators or by artificial intelligence (AI) programs. However, these techniques only show the static or passive aspect of a lesion, without the ability to explore further possible mechanisms triggering the formation, progression of coronary lesion, or inducing ischemia without anatomical stenosis. This study evaluates the possibility of an AI program based on Deep Learning (DL) algorithms to identify the vessel size, location, percentage of stenosis and calculate the arterial phase (AP).
Methods
A dataset of 226 angiograms of the Right Coronary Artery of patients¡¯ possible diagnosis of CAD was collected. These patients underwent a new angiographic recording technique. At first, the contrast was injected until the index coronary artery was completely filled. After the injection stopped, the blood in white color began moving in. The flow characteristics, direction, borders, and shape of the tip could be observed upon the black background of contrast. In order to assess the stenosis and the AP duration, all the angiographic images of the index artery needed to be included totally inside the screen so the computer program could capture and analyze the images. The AP started when the blood began to flow in and ended when all the contrast disappeared from the distal vasculatureIn this study, an AI program was built to detect the location and to quantify the severity of the stenosis, and calculate the duration of the AP. At first, for the detection of stenosis, Model 1 was built by the U-net combined Desenet121 to segmentation. Next, a centerline in the frames that had the vessels fully filled with contrast was delineated. Then with each 20 pixels of centerline, the diameter of each segment could be calculated and compared with each other in order to identify the location and quantify the stenotic area. For the calculation of the AP duration, a protocol was designed with the identification of two keyframes: (1) The first frame when the blood began to move in and (2) the final frame in which all contrast was completely washed out from the distal vasculature (Fig 1). At first, we applied the same architecture of model 1 to build the Carthete Guide segmentation model in order to identify the coronary artery ostium. Then the beginning of the AP (seen as blood in white moved in) was identified by the Convolutional Neural Network model with pixel shift at the coronary ostium. Finally, the segmentation model (model 1) was used again to identify the frame without contrast (ending frame of AP). We identified the first black image in each series of segmented images as the end of the AP.
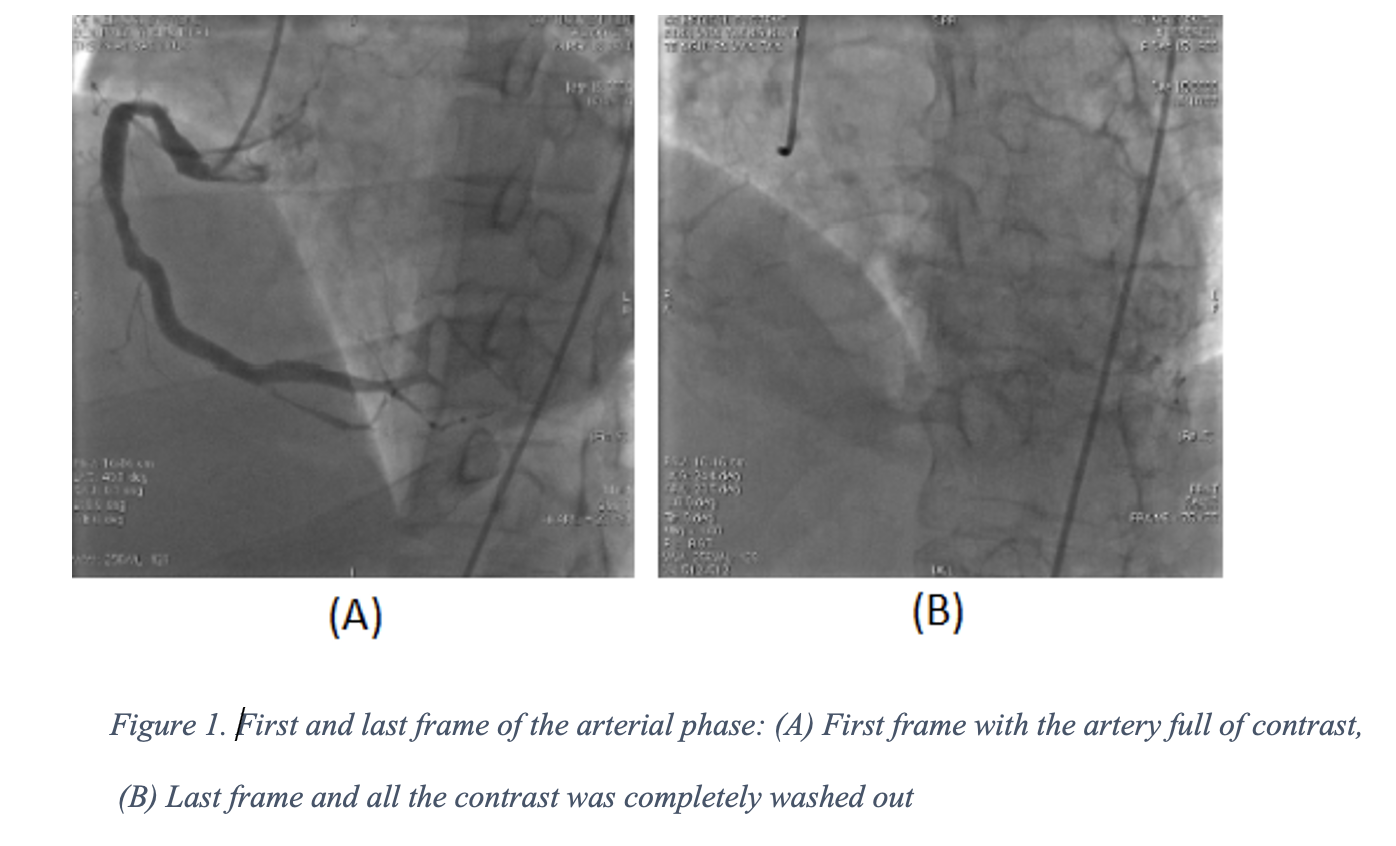
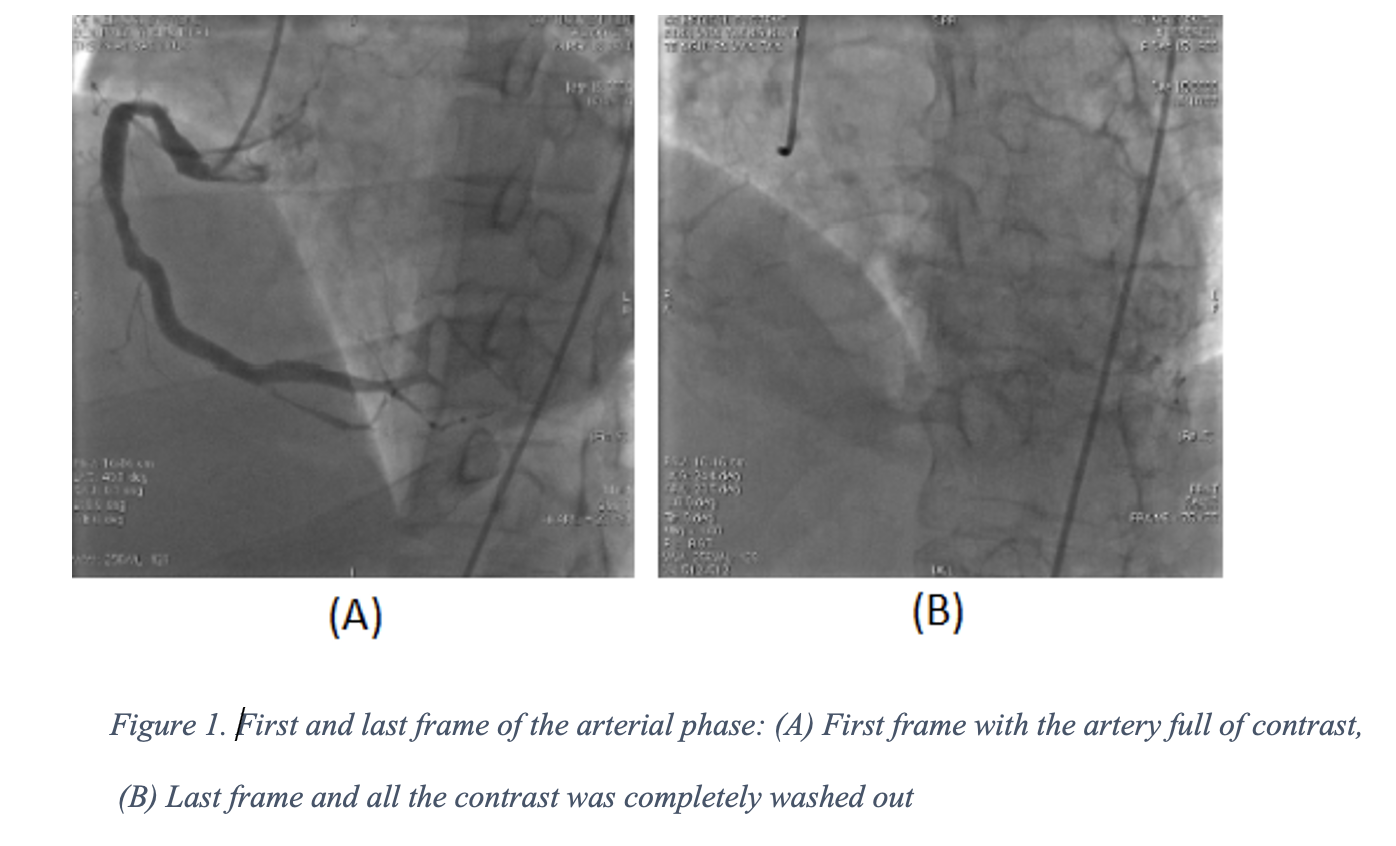
Results
Twenty-seven patients from the dataset met the inclusion criteria, with a mean age of 66.6 +/- 13 years and 62.9% of the male gender. If the AI protocol was programmed to search 6 frames with the highest contrast of each CAG, the results showed 99 locations of the lesion with >20% stenosis (Fig. 2). If the AI program searched only one frame with the highest contrast in the vessel, the AI program could detect a moderate lesion (25%-50% stenosis) in 4 cases (4.0 %), or a severe lesion (from 50% to 70%) in 8 cases (8%). At the same time, an interventional cardiologist reading showed no significant lesion in 9 (33.3 %) patients, one lesion in 13 (48.2%) patients, and two lesions at 5 (18.5%). In the calculation of the AP, the mean duration was at 1.84 +/- 0.44s. In patients with normal coronary angiography, the mean AP duration was 1.59 +/- 0.37s, while in patients with one lesion, the mean AP length was 1.96 +/- 0.42s (Fig 3). The mean difference in the AP values between the two groups was 0.37s (95% CI 0.034, 0.7; P= 0.03) which was statistically significant. Furthermore, The mean AP values in females were 1.61s compared with 1.97s of males, the difference was 0.36s (95% CI 0.013, 0.697; P= 0.04) (Fig 4). Our DL model has a root mean square error in predicting the AP which was 0.36s.
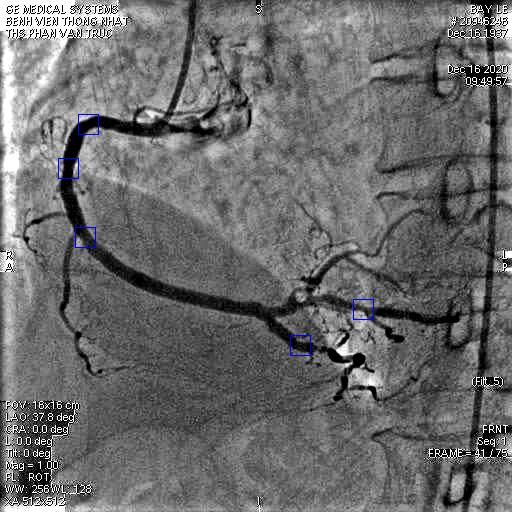
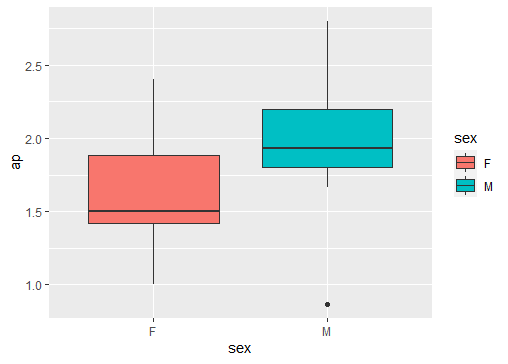
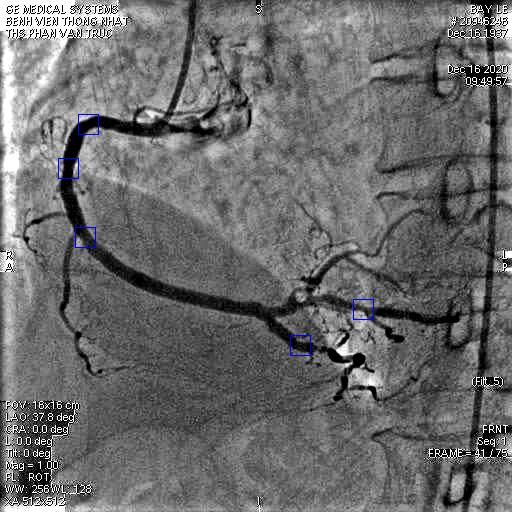
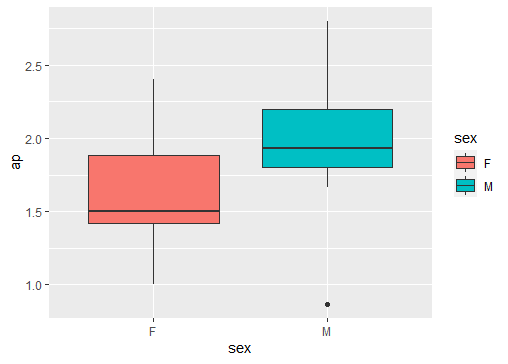
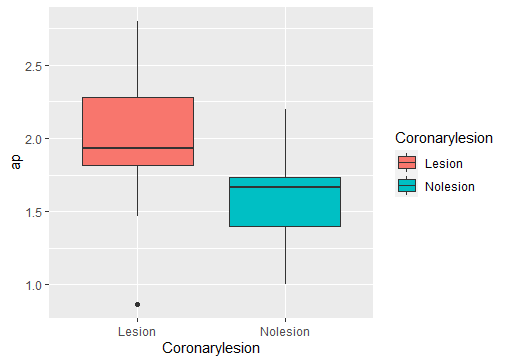
Conclusion
In the care of CAD patients, the AI program based on DL algorithms successfully identified the size of the artery, location, percentage of stenosis, and calculated the arterial phase (AP). The above information is important and should be validated with a larger number of patients. AI programs could be considered a potential marker for assessing coronary function in the improvement of care for cardiac patients.