Lots of interesting abstracts and cases were submitted for TCTAP 2021 Virtual. Below are accepted ones after thoroughly reviewed by our official reviewers. Don¡¯t miss the opportunity to explore your knowledge and interact with authors as well as virtual participants by sharing your opinion!
TCTAP A-044
Presenter
Jihoon Kweon
Authors
Jung-Eun Park1, Jihoon Kweon1, Do-Yoon Kang1, Pil Hyung Lee2, Jung-Min Ahn1, Soo-Jin Kang1, Duk-Woo Park1, Seung-Whan Lee1, Cheol Whan Lee1, Seong-Wook Park1, Seung-Jung Park1, Young-Hak Kim1
Affiliation
Asan Medical Center, Korea (Republic of)1, Seosan Jungang Hospital, Korea (Republic of)2
View Study Report
TCTAP A-044
Imaging: Intravascular
Deep Learning Segmentation of Lumen and Vessel on IVUS Images
Jung-Eun Park1, Jihoon Kweon1, Do-Yoon Kang1, Pil Hyung Lee2, Jung-Min Ahn1, Soo-Jin Kang1, Duk-Woo Park1, Seung-Whan Lee1, Cheol Whan Lee1, Seong-Wook Park1, Seung-Jung Park1, Young-Hak Kim1
Asan Medical Center, Korea (Republic of)1, Seosan Jungang Hospital, Korea (Republic of)2
Background
Intravascular Ultrasound (IVUS) is one of the most common imaging modality in the clinical field. However, due to the poor image contrast of ultrasound, it is very hard to clearly differentiate structures such as lumen and vessel areas on the image. As a result, it has still remained a challenging task even though state-of-the-art image processing technology has been developed. Recently, wide applications of deep learning have been reported for various medical imaging modalities like X-ray, computed tomography (CT), and magnetic resonance imaging (MRI). In this study, a novel deep learning method was proposed for the accurate segmentation of IVUS images.
Methods
118 IVUS imaging was conducted and collected for 101 patients who visited Asan Medical Center (AMC) in Nov. 2016. After excluding 14 IVUS imaging for the previous stenting, three radiographers with more than ten years of experience annotated lumen and vessel on 9715 frames. The proposed model created by modifying U-Net architecture had two decoders for shape and edges, respectively. The loss functions were dice coefficient loss and weighted dice coefficient loss for each decoder. Model performance was evaluated with Jaccard Index (JI), Hausdorff Distance (HD), and Percentage of Area Difference (PAD) were also considered besides Dice Coefficient (DC).
Results
Overall, deep learning models showed excellent performance for the segmentation of both lumen and vessel areas. the proposed model best performed for lumen segmentation indicating 0.9483 of DC, 0.9038 of JI, 4.2812 of HD, and 6.8784 of PAD for lumen segmentation. For vessel area, the proposed model showed 0.9642 of DC, which was comparable to that from DeeplabV3+.
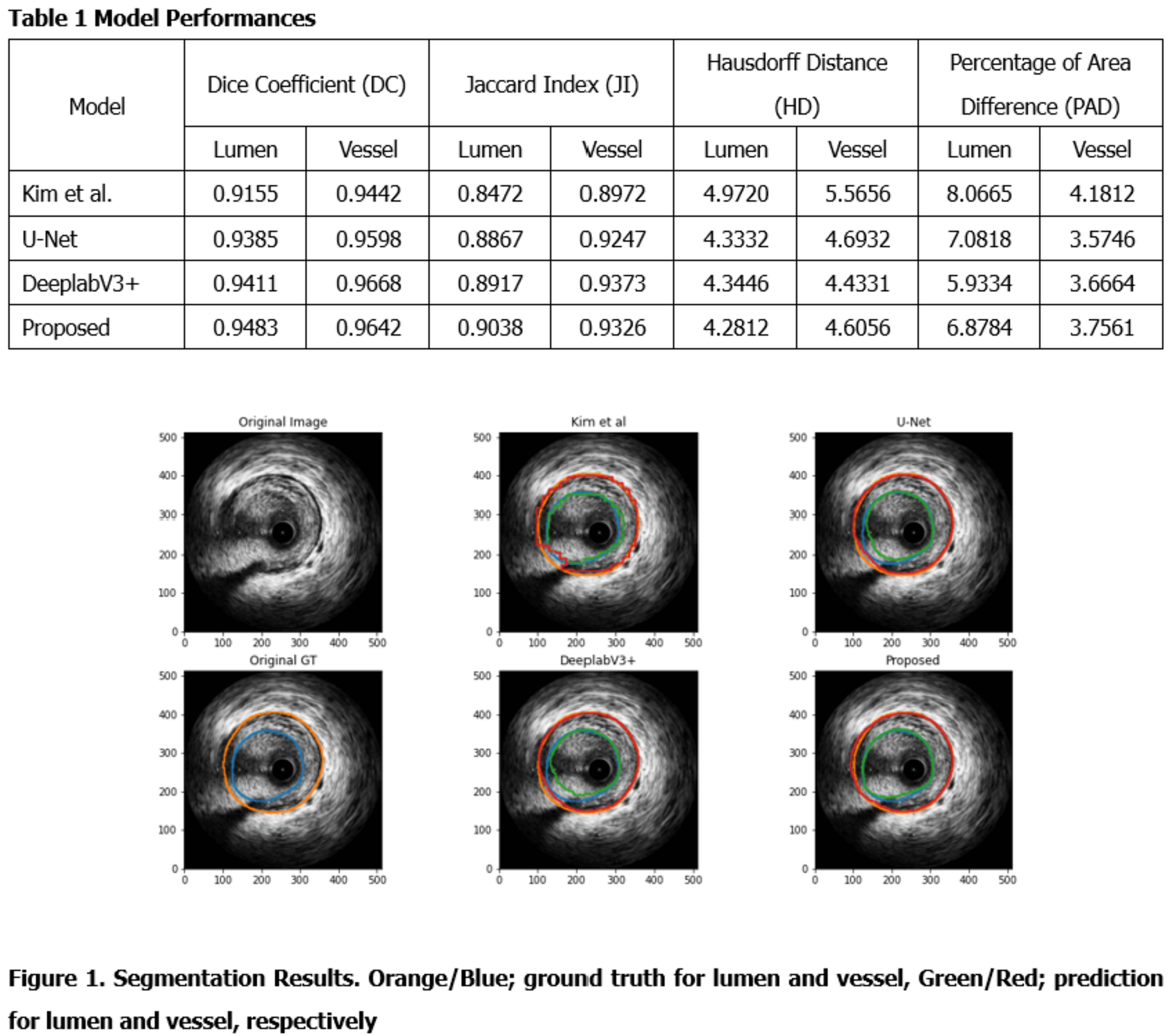
Conclusion
By applying deep learning segmentation, IVUS segmentation could be automated, thereby facilitating 3D modeling and fusion techniques with other imaging modalities.